报告时间:2021年6月9日13:00-15:00
报告地点:理科1号楼1126会议室
报 告 人:杨子超、仉尚航
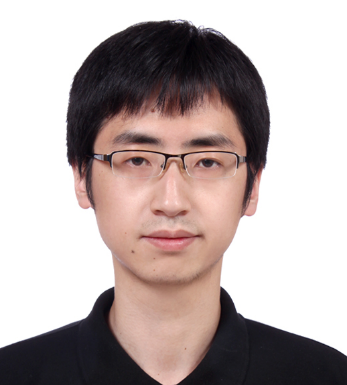
杨子超
Biography:
Zichao Yang’s research interests are in machine learning, deep learning and their applications in big data analytics, computer vision and natural language processing. He obtained Phd degree in Computer Science from CMU, and MPhil and BS from Chinese University of Hong Kong and Shanghai Jiao Tong University respectively. He has published dozens of papers in top ML/NLP conferences and has accumulated more than 6000 citations according to Google Scholar. He interned in Google DeepMind, Google Brain and Microsoft Research during his Phd. He is currently a machine learning researcher in Citadel Securities, focusing on machine learning research in financial industry.
Structural neural network models
Abstract:
Neural networks—the backbone of deep learning that have achieved great breakthroughs in vision and language—are often blamed for data inefficiency and lack of interpretability. In this talk, I will present my recent efforts to overcome these weaknesses by leveraging the structural property of data in designing neural network models. Specifically, I use the structural properties of data as a type of prior knowledge to inform and explain the computational structure of neural network models. The structure of data can act as a scaffold to guide and control the information flow in neural networks. With such type of constraints, neural networks are biased to uncover patterns in data automatically. I will present three examples in visual question answering, task-oriented dialogues and unsupervised models to demonstrate such kind of structural design works generally. Such models not only achieved start-of-the-art performance, but also were more explainable.
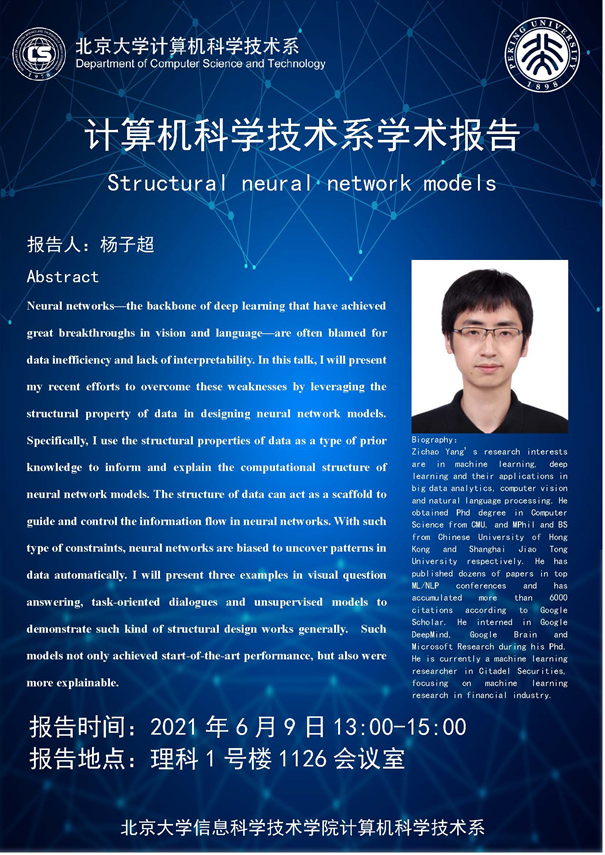
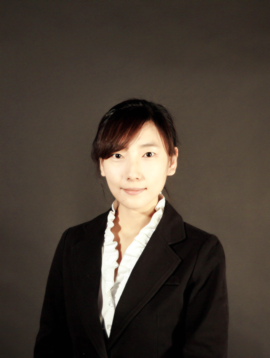
仉尚航
Biography:
Dr. Shanghang Zhang is a postdoc research fellow in the Berkeley AI Research Lab (BAIR), EECS, UC Berkeley. Her research focuses on generalized and efficient machine learning that can adapt to new domains and tasks using limited labels, with applications to IoT problems including smart traffic and intelligent manufacture, as reflected in her many publications on top-tier journals and conference proceedings, including NeurIPS, ICLR, ACM MM, TNNLS, TMM, CVPR, ICCV, and AAAI. She has been the author and editor of book “Deep Reinforcement Learning: Fundamentals, Research and Applications” published by Springer Nature. This book is selected to Annual High-Impact Publications in Computer Science by Chinese researchers and its Electronic Edition has been downloaded 45,000 times worldwide. Her recent work “Informer: Beyond Efficient Transformer for Long Sequence Time-Series Forecasting” has received the AAAI 2021 Best Paper Award. She was one of the “2018 Rising Stars in EECS, USA”. She has also been selected for the Adobe Academic Collaboration Fund, Qualcomm Innovation Fellowship (QInF) Finalist Award, and Chiang Chen Overseas Graduate Fellowship. Her research outcomes have been successfully productized into real-world machine learning solutions and filed 5 patents. Dr. Zhang has been the chief organizer of several workshops on ICML/NeurIPS, and the special issue on ICMR. Dr. Zhang received her Ph.D. from Carnegie Mellon University in 2018, and her Master from Peking University.
Towards More Generalized Machine Learning
Abstract:
Even though a great deal of existing work has been devoted to the field of machine learning, it still suffers from severe challenges: 1) Domain shift and novel categories of objects often arise dynamically in nature, which fundamentally limits the scalability and applicability of deep learning models to handle this dynamic scenario when labeled examples are not available. 2) Since real-world data usually varies over different environments and has a long-tailed distribution, it is prohibitively expensive to annotate enough data to cover all these variances. However, existing deep learning models usually lack generalization capability and fails to generalize to the out-of-distribution data with limited labels. In this talk, I will introduce my research on how to address these challenges by building generalized machine learning systems that can automatically adapt to new domains, tasks, and dynamic environments with limited training data. Specifically, I will talk about a series of my research on both theoretical study and algorithm design from three aspects: 1) Generalize to new domains; 2) Generalize to new categories; 3) Generalized and efficient machine learning for IoT applications, including intelligent transportation and manufacture, which promotes the landing of AI in the real world. Especially, I will discuss the exploration of brain cognition mechanism to develop generalized machine learning that can adapt to new domains and modalities with limited labels.
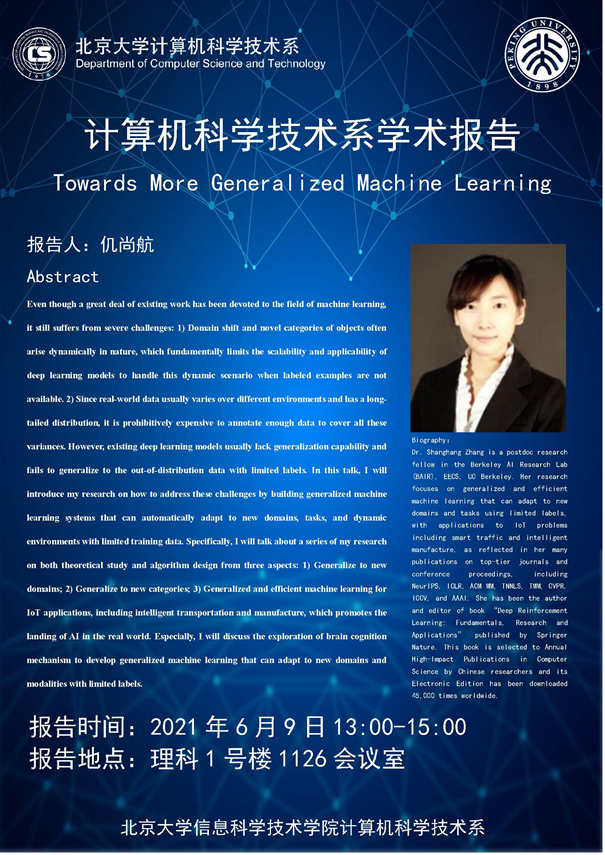
欢迎参加,现场交流!